|
Genes underlying normal skin variations
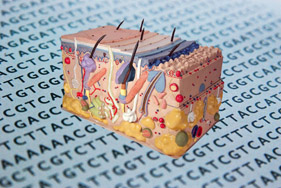
Skin is the human body's largest organ and the first to contact various environments. Many normal skin phenotypes have substantial variations both within and between populations, as a result of adaptation to the local environment through natural selection. For decades, skin research has been focusing on clinically relevant skin diseases, while normal skin phenotypes are generally understudied. These normal skin phenotypes, exemplified by skin pigmentation, have high heritability. A well-designed genome-wide association scan (GWAS) should be able to identify the underlying genetic variants.
Collaborating with dermatologists, we design quantitative methods to measure normal skin phenotypes. We then collaborate with the Taizhou longitudinal cohort to perform collections of both phenotype and genotype data. After performing the GWAS, we further integrate a range of state-of-the-art genomic tools to prioritize a set of candidate genes, based on both statistical analysis and biological relevance, for further functional validation. We have already published our GWAS results of hair straightness (Wu et al., 2016), petaloid toenail (Zhang et al., 2016), and skin barrier function (Zhang et al., 2017). More GWAS work of further normal skin phenotypes will be seen in the near future.
Gene-environment interaction and extrinsic skin aging
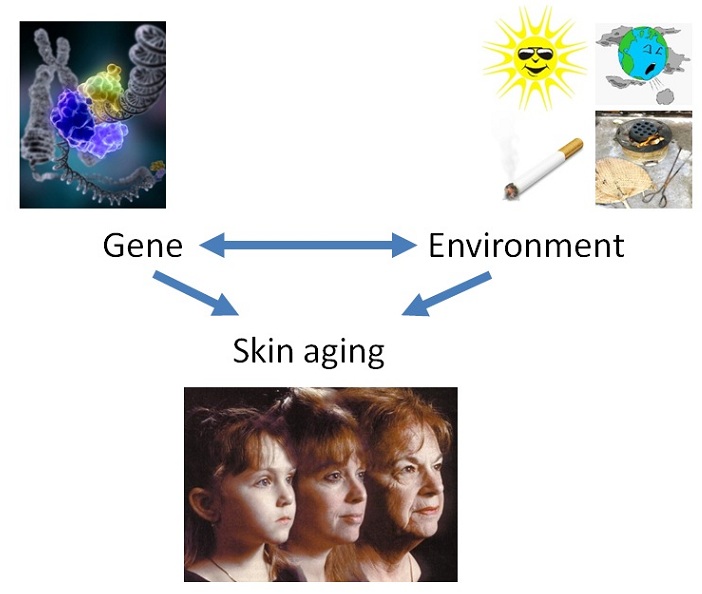
Most of the skin phenotypes are affected by both genetic and environmental factors. Taking the example of skin aging related phenotypes, such as wrinkle and pigmented spots, they have been linked to chronic exposure to solar radiation and cigarette smoke, and most recently, to industrial and traffic-related pollution. However, skin aging varies strikingly among individuals and among ethnic populations. The biological mechanism behind extrinsic skin aging could be complicated and involves interactions between genes and environments. Therefore it is extremely important to explore both genetic and environmental factors, as well as the interaction between them, when studying skin phenotypes.
In the Taizhou longitudinal cohort, we firstly reported the link between skin aging (particularly wrinkles) and the use of fossil fuel (Li et al., 2015). We further confirmed that indoor PM2.5 is directly associated with wrinkles (Ding et al., 2017). We also found that the gas pollutant (NO2 and SO2) are associated with skin aging signs, particularly lentigines on cheeks, suggesting PM and gas might be affecting skin aging from different mechanisms (Huls et al., 2016). Using candidate gene approaches, we identified genetic variants associated with skin aging signs in Chinese, suggesting different genetic mechanisms of skin aging in different ethnic groups (Gao et al., 2017). Further work on the GWAS of skin aging signs, as well as the gene-environment interaction on skin aging will be seen in the near future.
Adaptation of skin-related traits in human evolution
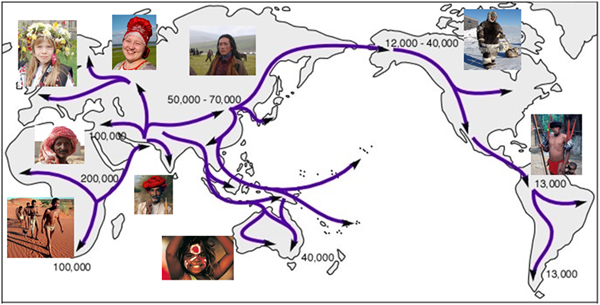
Having originated from Africa more than 100 thousand years ago, modern humans quickly occupied different environmental niches all over the world in a relatively short period of time. Local adaptation has been shaping us into an extremely diverse species with a great deal of phenotypic variation. Skin, as the first contact to the environment has experienced extensive adaptive selection in the past tens of thousands years. The lightened skin color in Caucasians, for example, has been widely seen as an adaptation to compensate vitamin D deficiency. It was caused by a number of genetic variants independently. Many other genes affecting normal skin phenotypes are also subject to strong selective adaptation, but the mechanisms and the evolutionary significance remain to be investigated.
Our previous finding indicated that a strongly selected variant EDARV370A appeared in current day central China more than 30,000 years ago, and swept to high frequency in a short period of time because of its selective advantage. We found that EDARV370A is associated with several phenotypic changes of epidermal appendages, including an increased density of sweat glands. This could be a crucial feature in human evolution, as superior thermoregulation is thought to be one of the main advantages in prehistoric hunting (Kamberov et al., 2013). We also showed a clear pleiotropic effect of EDARV370A, as it is associated with hair curliness, hair thickness, incisor shoveling, chin shape and earlobe shape (Tan et al., 2013, Tan et al., 2014, Wu, et al, 2016; Peng et al., 2016). We are currently working on selected variants at ABCC11, OCA2 and other skin-related genes, to explore their potential evolutionary significance.
Quantitative and high-throughput phenotyping through image analysis
Accurate and efficient methods to quantify phenotype are vastly important for genome-wide scans on complex traits. Since large sample sizes are required for such studies, automatic phenotyping is highly desirable. Using image analysis, we have already developed semi-automatic pipelines for sweat gland and hair counting, and for wrinkles and pigmented spots detection. We are further developing deep learning based model for the image analysis to achieve better accuracy.
Systematic analysis including transcriptome and epigenome data
Genome-wide expression differentiation and epigenome-wide analysis have been effectively applied to illustrate the genetic variations in different skins. On top of our existing data (skin phenotypes, environmental factors, and genomic data), it would be extremely valuable to further collect the transcriptome and epigenome data in the dermatogenomics research, as these are the critical intermediate layers connecting the gene-environment-phenotype network. We are currently making this expansion, as we are able to secure the skin biopsy samples through collaboration with dermatologists. The systematic network analysis combining different omics data will bring new knowledge in skin research.
Interplay between skin microbiome and skin phenotypes
Skin microbiome plays crucial roles in skin health. While the standard practice of gut microbiome studies is relatively mature, the standard practice of skin microbiome studies is still in its infancy. By collaborating with the pioneering laboratories in the field of skin microbiome, we aim to establish the correlations between skin microbiome and skin phenotypes. We can further test the causality by carrying out interfering experiments on the skin microbiota.
Prediction models based on phenotype-phenotype correlations
For the common traits we are studying, it would be ideal if prediction models can be made to infer the traits/phenotypes - as what has already been done on the eye color. However, for many common traits, it is not practical to build prediction model based on genetic information alone. Since we can find significant amount of correlations between phenotypes - and there are often biological explanations behind such correlations - we will be able to improve the prediction model by taking advantage of the phenotype correlations. For example, our preliminary results showed that facial phenotype information can help improve the prediction power of certain diseases or health status. We are further exploring this area by carrying out a more systematic study collecting a large amount of phenotypes (including image and physiology phenotypes, as well as the molecular phenotypes) at the same time.
|
|
|