Andrew E. Teschendorff,Andrew P. Feinberg. Statistical mechanics meets single-cell biology.
Nat Rev Genet.
(2021) Click here for more infoTeschendorff AE. Avoiding common pitfalls in machine learning omic data science.
Nat Material.
(2019) Click here for more infoZheng SC, Breeze CE, Beck S, Teschendorff AE. Identification of differentially methylated cell types in epigenome-wide association studies.
Nat Methods.
(2018) Click here for more infoTeschendorff AE, Relton CL. Statistical and integrative system-level analysis of DNA methylation data.
Nat Rev Genet
(2018) Click here for more infoTeschendorff AE, Enver T. Single-cell entropy for accurate estimation of differentiation potency from a cell's transcriptome
Nat Commun
(2017) Click here for more infoZheng SC, et al. Correcting for cell-type heterogeneity in epigenome-wide association studies: revisiting previous analyses
Nat Methods
(2017) Click here for more infoYang Z, et al. Correlation of an epigenetic mitotic clock with cancer risk
Genome Biol
(2016) Click here for more infoZheng SC, Widschwendter M, Teschendorff AE. Epigenetic drift, epigenetic clocks and cancer risk
Epigenomics
(2016) Click here for more infoTeschendorff AE, et al. DNA methylation outliers in normal breast tissue identify field defects that are enriched in cancer
Nat Commun
(2016) Click here for more infoTeschendorff AE, et al. Correlation of Smoking-Associated DNA Methylation Changes in Buccal Cells With DNA Methylation Changes in Epithelial Cancer
JAMA Oncol
(2015) Click here for more infoYuan T, et al. An integrative multi-scale analysis of the dynamic DNA methylation landscape in aging
PLoS Genet
(2015) Click here for more infoJones A, et al. Role of DNA methylation and epigenetic silencing of HAND2 in endometrial cancer development
PLoS Med
(2013) Click here for more infoWest J, Widschwendter M, Teschendorff AE. Distinctive topology of age-associated epigenetic drift in the human interactome
Proc Natl Acad Sci U S A.
(2013) Click here for more infoTeschendorff AE, et al. A beta-mixture quantile normalization method for correcting probe design bias in Illumina Infinium 450 k DNA methylation data
Bioinformatics
(2013) Click here for more infoTeschendorff AE, et al. Epigenetic variability in cells of normal cytology is associated with the risk of future morphological transformation
Genome Med
(2012) Click here for more infoTeschendorff AE, Zhuang J, Widschwendter M. Independent surrogate variable analysis to deconvolve confounding factors in large-scale microarray profiling studies
Bioinformatics
(2011) Click here for more infoTeschendorff AE, et al. Age-dependent DNA methylation of genes that are suppressed in stem cells is a hallmark of cancer
Genome Res
(2010) Click here for more info
Research Focus
Computational Systems Biology Analysis of Single-Cell data

We are developing novel computational and statistical methods to help analyse and better interpret single-cell omic data, with a particular focus on systems biological aspects.
Epigenomics of Ageing, Stem Cells and Cancer

We are exploring how informative DNA methylation changes that accrue in normal cells as a function of age and exposure to risk factors are for predicting cancer risk.
Cell-type heterogeneity and deconvolution for EWAS
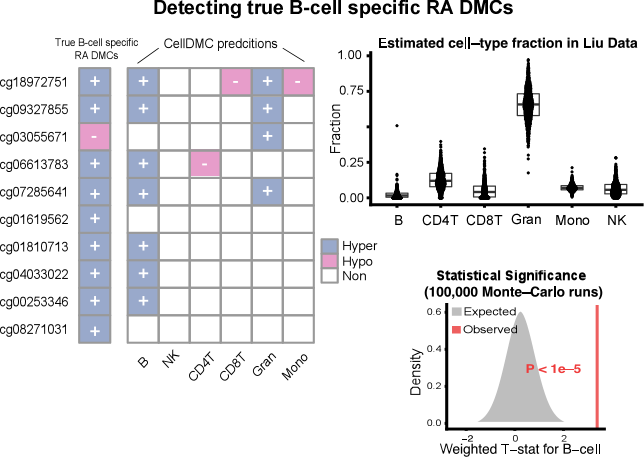
We are exploring and developing statistical cell-type deconvolution methods to dissect cell-type heterogeneity aimed at epigenome studies performed in general complex tissues.
Cancer System-Omics and Network Physics

We use innovative strategies drawing on methods from graph theory/network science/signal processing to analyse complex and high-dimensional multi-omic data, with a view to elucidate the systems-biological principles underlying diseases like cancer.
News and Article
Recent News:
Recent Article:
Designed and Coded by Yuan Tian